JUMP TO:
3D tissue imaging and cytometry
Goal:
Understand the kidney in health and disease via 3D imaging and cytometric analyses.
.jpeg)
The following image types within this technology are available in the Kidney Tissue Atlas:
- Composite 3D 8-channel immunofluorescence image volume
3D volume completely represented as a stack of individual, 8-channel images. Every focal plane image and every channel can be independently inspected.
- Composite max projection of 8-channel immunofluorescence image volume
8-channel volume combined into a single maximum projection; composite image consists of 8 channels.
- RGB max projection of 2-channel (autofluorescence and second harmonic generation) image volume
Projection of 3D volume collected prior to labeling; channels cannot be controlled.
- RGB max projection of 8-channel immunofluorescence image volume
8-channel volume combined into a single maximum projection and converted to RGB color space.
Protocol(s)
Metadata standards
CODEX (CO-detection by InDEXing)
Goal:
To build a library of high-resolution phenotypical maps of kidney biopsies with anchor, immune, and functional markers for in situ spatial analysis at single cell resolution in normal and pathological conditions.

Protocol(s)
- (UCSF) Sample Preparation and Staining Experiment Protocol
- (UCSF) Imaging Setup and Processing Experiment Protocol
Metadata standards
Imaging mass cytometry
Goal:
To generate high-dimensional spatially preserved data at single cell resolution to define the cellular milieu and cell-cell interactions in normal and injured kidneys.

Protocol(s)
Metadata standards
MULTIMODAL IMAGING MASS SPECTROMETRY
Goal:
Our multimodal molecular imaging pipeline combines imaging mass spectrometry (IMS), Matrix-assisted laser desorption/ionization (MALDI) IMS, generates molecular images of metabolites and lipids with high spatial fidelity, showing localization to different functional tissue units (FTU) in the kidney. Analytes can be identified with a combination of accurate mass measurements (<1 ppm error) and complimentary liquid chromatography (LC)-based fragmentation experiments. By integrating this technology with advanced machine learning, Kidney Precision Medicine Project (KPMP) researchers will enable a more comprehensive understanding of the molecular and structural drivers of acute kidney injury (AKI) and chronic kidney disease (CKD).

Protocol(s)
- Autofluorescence Microscopy QC for Multimodal Molecular Imaging
- Kidney Functional Tissue Unit (FTU) Segmentation
Protocols used by Vanderbilt University TIS for Multimodal Molecular Imaging of Human Kidney Biopsies
Overall Pipeline:
- VU TIS Multimodal Molecular Imaging Pipeline for KPMP Biopsy Interrogation (not yet available)
- Tissue Screening and Assessment (not yet available)
Sample Interrogation Assays:
- Autofluorescence Microscopy QC
- Autofluorescence Microscopy Data Collection
- MALDI IMS Matrix Deposition
- MALDI IMS Data Collection
- LC-MS/MS Lipidomics Data Collection
- Post-IMS AF Microscopy
- PAS Staining
Data Pre-processing and Analysis
- Multimodal Image Registration
- MALDI IMS Data Pre-processing
- Functional Tissue Unit Segmentation
- Analyte Annotation
- Quality Control (not yet available)
Metadata standards
- Not yet available
JUMP TO:
- Regional transcriptomics
- Single-cell RNA-seq
- Single-nucleus RNA-seq
- Single-nucleus (sn) RNA-seq + snATAC-seq (10X Multiome)
- Spatial transcriptomics
Single-nucleus RNA-seq
Goal:
Identify cell types and states associated with normal and injured kidney functions using gene expression profiling. Identify marker genes for cell type/states and any proportion shifts underlying pathology.

Protocols:
- Preparation
- Isolation of single nuclei from solid tissues
- snDrop-seq
- 10x Genomics snRNA sequequencing for TRA
Metadata standards
Single-cell RNA-seq
Goal:
Empirically derive cell subtypes and cell-type-specific gene expression profiles.
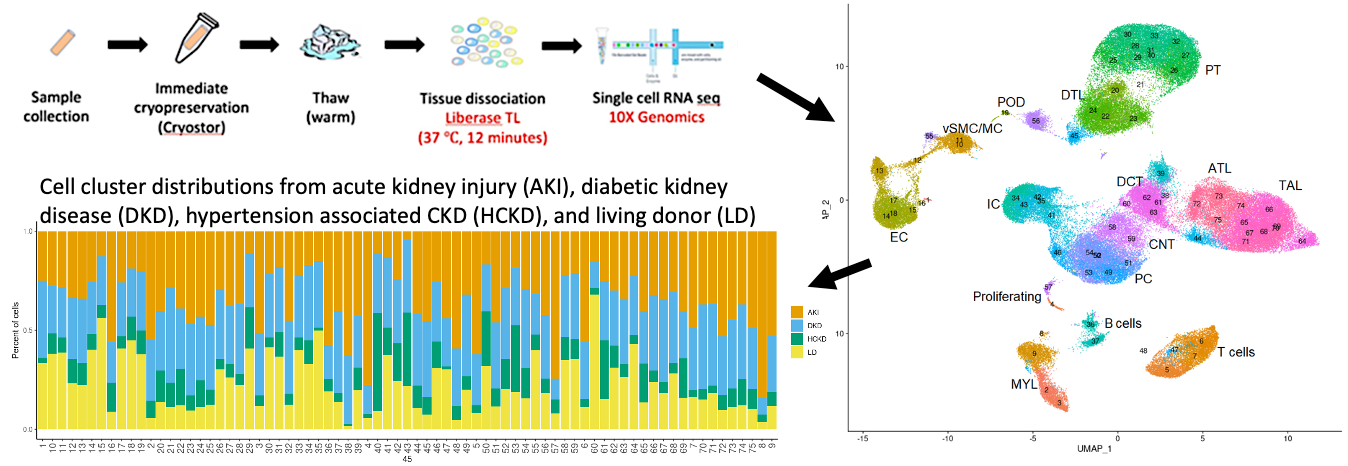
Protocol(s)
Metadata standards
Regional transcriptomics
Goal:
Generate deep transcriptomic signatures from nephron segments defined spatially by antibody staining using laser microdissection.
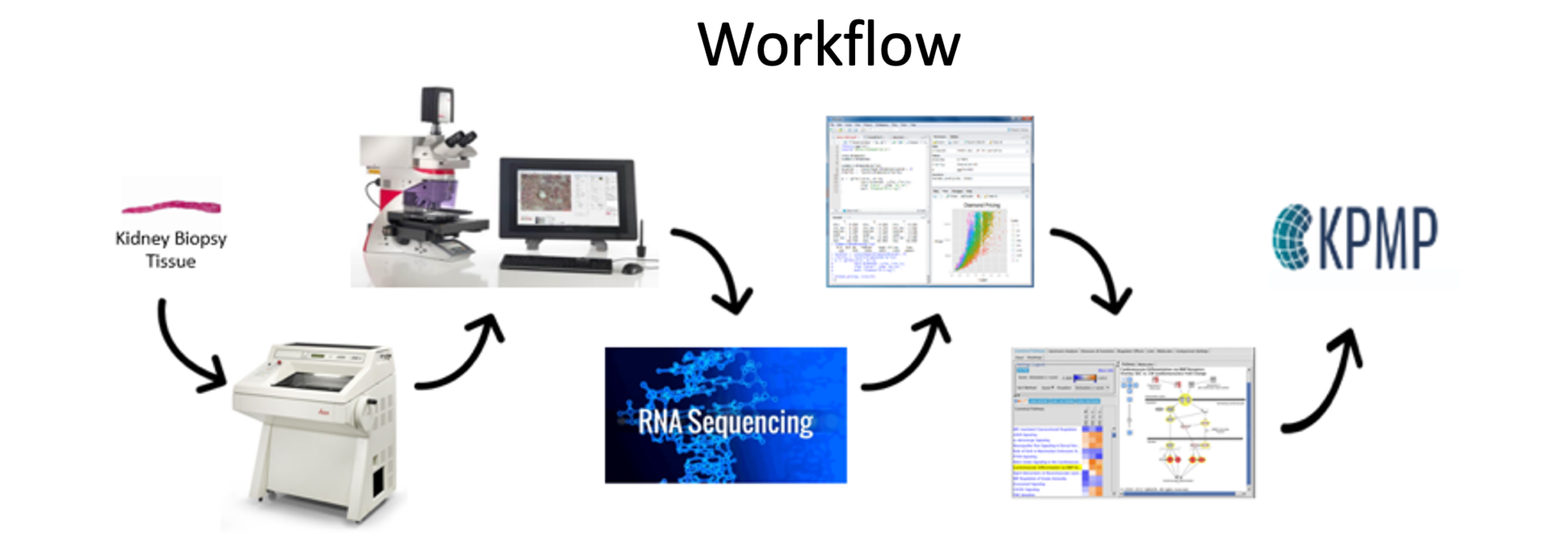
Protocol(s)
Metadata standards
Spatial transcriptomics
Goal:
Capture whole transcriptome mRNA expression with localization to kidney cells and structures.
.jpg)
Protocol(s)
Metadata standards
- Not yet available
Single-nucleus (sn) RNA-seq + snATAC-seq (10X Multiome)
Goal:
10X Genomics multiome protocol generates a molecular atlas of the human kidney with comprehensive cell types and minimal processing artifacts. 10X Multiome snATAC-seq + Gene Expression assay allows measurements of chromatin accessibility and transcription from the same cell. This allows direct insights into putative transcription factors or SNPs associated with open chromatin and impact on gene expression.
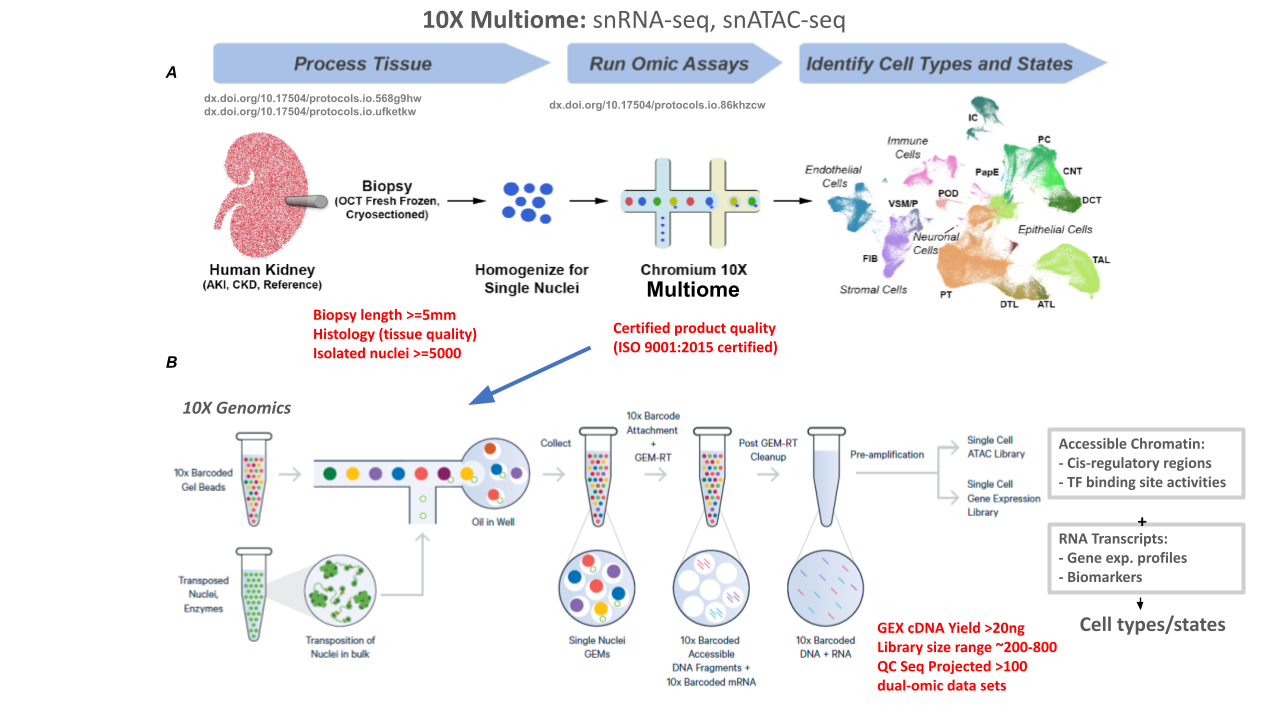
PROTOCOLS:
METADATA STANDARDS
JUMP TO:
Regional proteomics
Goal:
Characterize the kidney proteome in health and disease to identify protein markers that reflect each segment, matrix, and cell type contained in the tissue.

Protocol(s)
Metadata standards
- Not yet available
JUMP TO:
Spatial metabolomics
Goal:
Localize the small metabolite markers in tissue sections from human kidneys.

Protocol(s)
- Matrix-Assisted Laser Desorption/Ionization-Mass Spectrometric Imaging (MALDI-MSI)
- Tissue Preparation for Spatial Metabolomics
- Optical Image Collection
Metadata standards
Spatial lipidomics
Goal:
Localize the lipid markers in tissue sections from human kidneys.
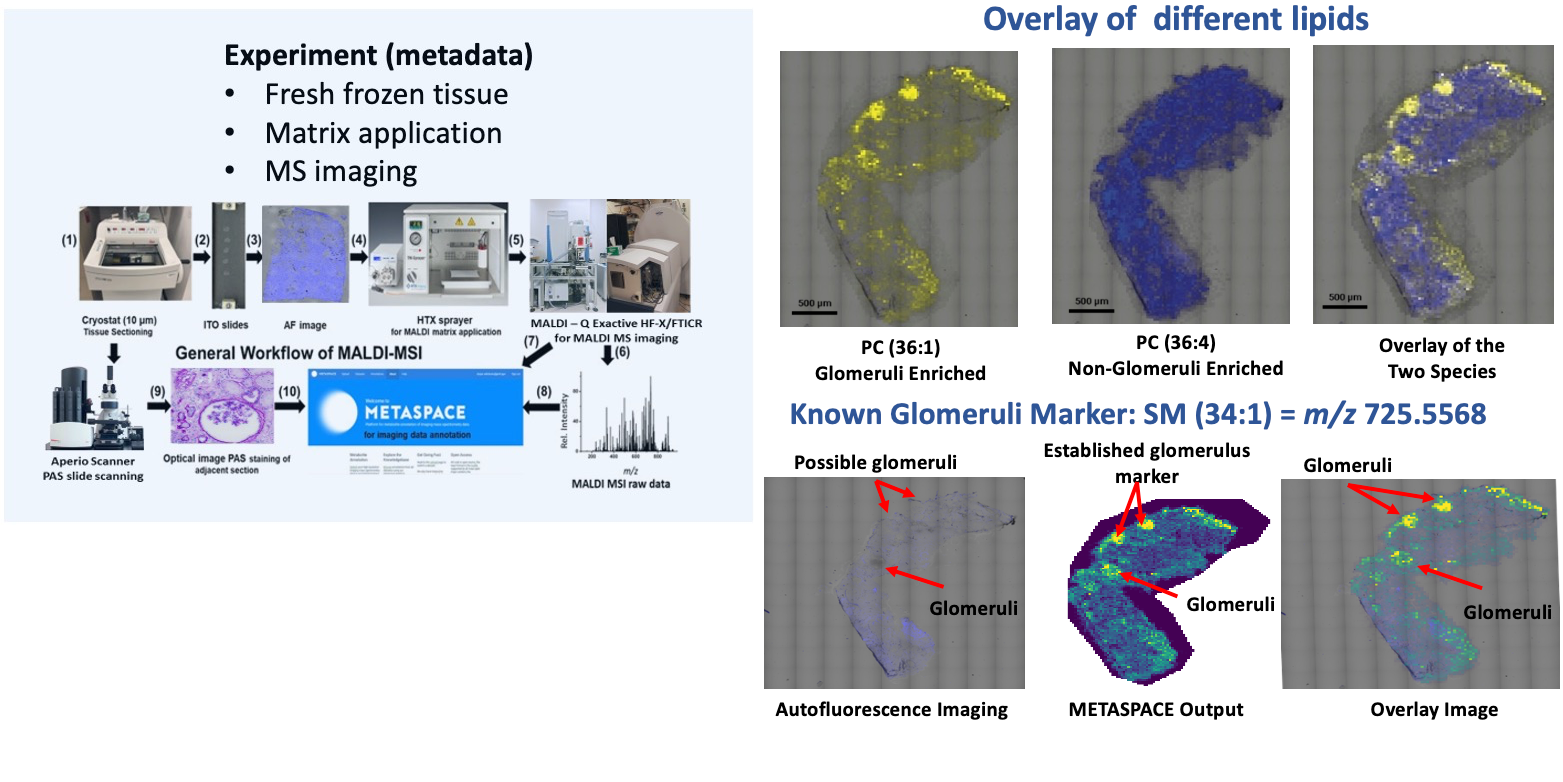
Protocol(s)
Metadata standards
Spatial N-glycomics
Goal:
Localize the N-glycan markers in tissue sections from human kidneys

Protocol(s)
Metadata standards
JUMP TO:
CUT&RUN
Goal:
To obtain genome-wide profiles of histone post-translational modifications that define chromatin states of healthy and injured/repairing kidney tissue using Cleavage Under Targets and Release Using Nuclease (CUT&RUN). This technology enables the interrogation of chromatin profiles with limited tissue, such as kidney biopsies.
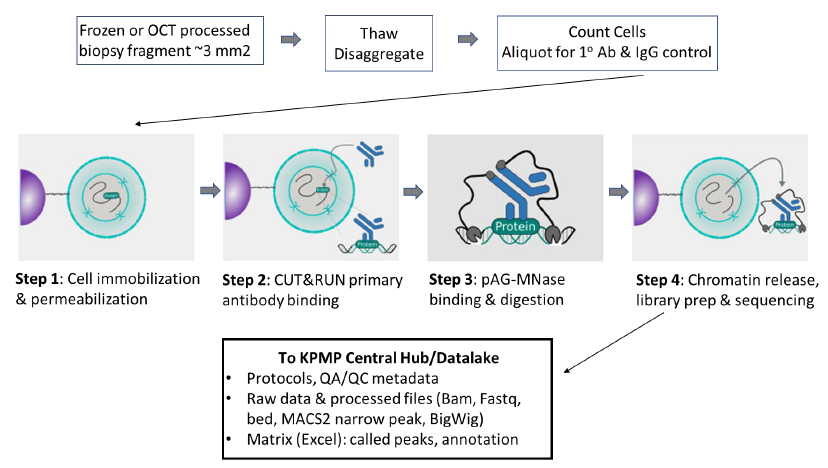
PROTOCOLS:
METADATA STANDARDS
DNA METHYLATION SEQUENCING
Goal:
The goal of this technology is to uncover methylation signatures of kidney sub-segments that are key determinants of kidney disease progression. This work will result in a comprehensive set of methylation patterns and genotypes to map back to a kidney atlas. This will allow the characterization of methylation signatures of over 20 million CpG sites in individual kidney compartments from both nephrectomies and biopsies using laser microdissection (LMD) methodology with downstream whole-genome bisulfite-sequencing (WGBS).
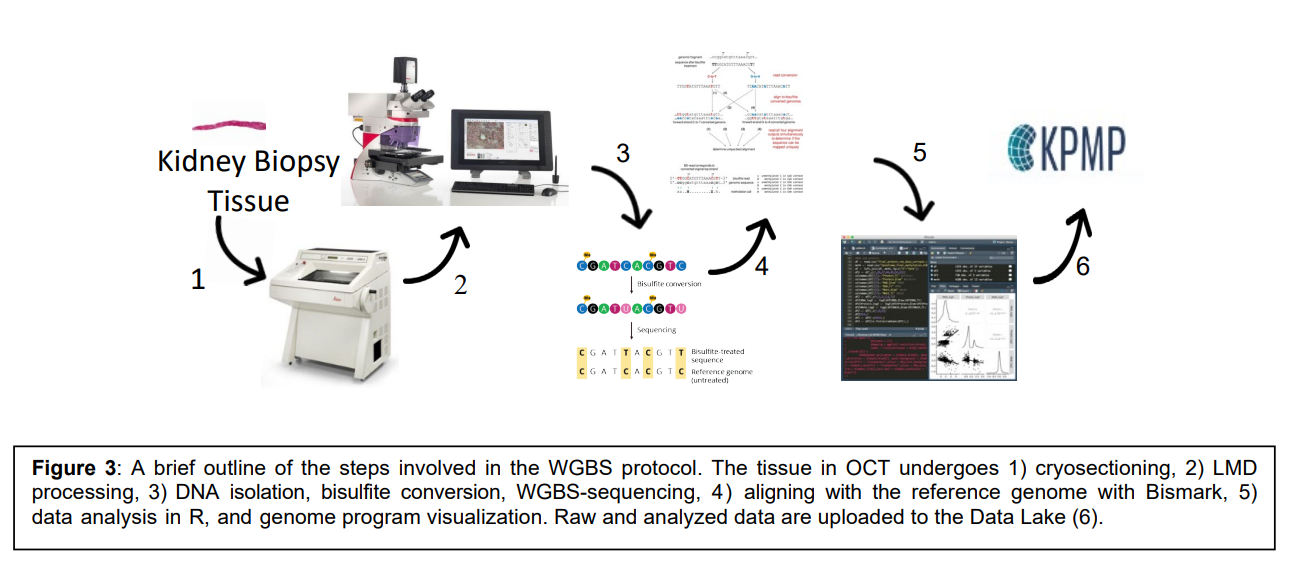
Workflow: 1) Cryosectioning, 2) LMD processing, 3) DNA isolation, bisulfate conversion, WGBS-sequencing, 4) aligning with the reference genome with Bismark, 5) data analysis in R, and genome program visualization, 6) raw and analyzed data are uploaded to the KPMP data lake.
PROTOCOLS:
METADATA STANDARDS
JUMP TO:
3D tissue imaging and cytometry
Goal:
Understand the kidney in health and disease via 3D imaging and cytometric analyses.
.jpeg)
The following image types within this technology are available in the Kidney Tissue Atlas:
- Composite 3D 8-channel immunofluorescence image volume
3D volume completely represented as a stack of individual, 8-channel images. Every focal plane image and every channel can be independently inspected.
- Composite max projection of 8-channel immunofluorescence image volume
8-channel volume combined into a single maximum projection; composite image consists of 8 channels.
- RGB max projection of 2-channel (autofluorescence and second harmonic generation) image volume
Projection of 3D volume collected prior to labeling; channels cannot be controlled.
- RGB max projection of 8-channel immunofluorescence image volume
8-channel volume combined into a single maximum projection and converted to RGB color space.
Protocol(s)
Metadata standards
CODEX (CO-detection by InDEXing)
Goal:
To build a library of high-resolution phenotypical maps of kidney biopsies with anchor, immune, and functional markers for in situ spatial analysis at single cell resolution in normal and pathological conditions.

Protocol(s)
- (UCSF) Sample Preparation and Staining Experiment Protocol
- (UCSF) Imaging Setup and Processing Experiment Protocol
Metadata standards
Imaging mass cytometry
Goal:
To generate high-dimensional spatially preserved data at single cell resolution to define the cellular milieu and cell-cell interactions in normal and injured kidneys.

Protocol(s)
Metadata standards
MULTIMODAL IMAGING MASS SPECTROMETRY
Goal:
Our multimodal molecular imaging pipeline combines imaging mass spectrometry (IMS), Matrix-assisted laser desorption/ionization (MALDI) IMS, generates molecular images of metabolites and lipids with high spatial fidelity, showing localization to different functional tissue units (FTU) in the kidney. Analytes can be identified with a combination of accurate mass measurements (<1 ppm error) and complimentary liquid chromatography (LC)-based fragmentation experiments. By integrating this technology with advanced machine learning, Kidney Precision Medicine Project (KPMP) researchers will enable a more comprehensive understanding of the molecular and structural drivers of acute kidney injury (AKI) and chronic kidney disease (CKD).

Protocol(s)
- Autofluorescence Microscopy QC for Multimodal Molecular Imaging
- Kidney Functional Tissue Unit (FTU) Segmentation
Protocols used by Vanderbilt University TIS for Multimodal Molecular Imaging of Human Kidney Biopsies
Overall Pipeline:
- VU TIS Multimodal Molecular Imaging Pipeline for KPMP Biopsy Interrogation (not yet available)
- Tissue Screening and Assessment (not yet available)
Sample Interrogation Assays:
- Autofluorescence Microscopy QC
- Autofluorescence Microscopy Data Collection
- MALDI IMS Matrix Deposition
- MALDI IMS Data Collection
- LC-MS/MS Lipidomics Data Collection
- Post-IMS AF Microscopy
- PAS Staining
Data Pre-processing and Analysis
- Multimodal Image Registration
- MALDI IMS Data Pre-processing
- Functional Tissue Unit Segmentation
- Analyte Annotation
- Quality Control (not yet available)
Metadata standards
- Not yet available